- June 28, 2023
- Posted by: Abishek Balakumar
- Category: Data & Analytics
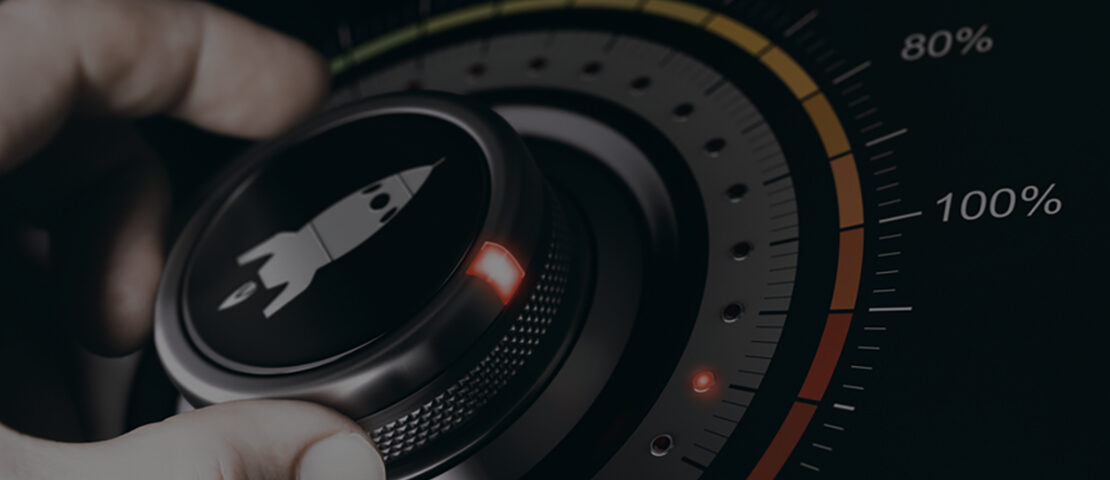
McKinsey’s report, ‘The Data-Driven Enterprise of 2025’, points out how though organizations apply data-driven approaches such as predictive analytics and AI-driven automation, it is still sporadic, ineffective, and time-consuming. By 2025, all employees will leverage data more uniformly using innovative data techniques that would help solve problems faster.
This will help to effect continuous improvement in performance and create differentiated experiences for customers and employees. It will also enable accelerated development of innovative new solutions.
McKinsey also identifies the current challenges to optimizing data sources as
- Limited capabilities of legacy technologies
- Challenges in modernizing the architecture.
- Demand for high computational resources for real-time processing jobs
This results in only a small part of the data from connected devices being leveraged. As companies balance speed and computational intensity, they are unable to perform complex analyses or implement real-time use cases.
Getting the right data technologies to ingest, process, analyze, and visualize in real-time is going to be a game-changer in improving decision-making, enhancing customer experience, and accelerating growth.
Improved Decision Making
Real-time data is critical for conducting real-time analytics, which helps with faster decision-making. Data is collected from a variety of sources, including sensors, databases, operational systems, cameras, and social media feeds with minimal delay and processed and analyzed quickly. They could be alerts and notifications or inputs from user behavior.
Real-time data can be of two types:
- Event Data: The generation of a collection of data points based on well-defined conditions within a system.
- Stream Data: The continuous generation of a large volume of data without any identifiable beginning or end.
Easy access to data in real-time data enables a quick drawing of insights to make informed decisions and be responsive as events unfold. It helps with capturing trends, both past, and present, and can be analyzed in real-time to decide on the next course of action.
Some of the benefits of real-time data include
Being Proactive
In the absence of real-time data, there is a lag between insights and responses. This reactive approach can prove costly, resulting in losing customers or production-related issues escalating. Real-time data analytics allows enterprises to proactively approach developments and respond appropriately.
Enhance Customer Experience
Visibility and transparency have become key in several client-business relationships. It helps improve decision-making based on project status and enhances customer experience and retention. Responding to customer requirements and empowering them with information in real-time further strengthens the relationship between the two.
To know more about how Indium can help you, please check out more.
Unify Data
Different teams end up creating data silos to suit their requirements. This can distort the view when making strategic decisions at the enterprise level and delay the process. A cloud-based data streaming solution helps to provide a unified view in real-time while allowing different teams access to secure and permission-based data they need to make decisions for their department.
Improve Operational Excellence
Real-time data allows you to manage your organization’s assets proactively. It lets you plan downtimes for maintenance and repair, improves the life of the assets, and take timely steps to replace, where needed, with minimum disruption to operations. This naturally leads to a better quality of products and services and improved profit margins as it lowers overheads.
Striim Power For Real-time Data Analytics
The Striim unified real-time data integration and streaming platform unifies data across multiple sources and targets. It offers built-in adapters and supports more than 125 sources and targets, enabling the management of multiple data pipelines in a Striim cluster. Striim 4.1 offers features such as OJet to let customer applications read multi-terabytes of data per day and a high-performance Oracle Change Data Capture (CDC) reader. It also sends real-time alerts and notifications to identify emerging workload patterns and facilitates collaboration between developers and database administrators.
Striim users can build smart real-time data pipelines quickly for streaming large volumes of events daily. It is scalable and secure, and the features are highly available. It is easy to maintain and allows the rapid adoption of new cloud models, infrastructure modernization, and digitalizing legacy systems.
Striim enables data integration using a streaming-first approach, supporting incremental, real-time views in the cloud database and the streaming layer. It includes Streaming SQL to facilitate real-time analytics of data, as also train machine learning models in real-time.
Business analysts, data scientists, and data engineers can use Streaming SQL to build data pipelines quickly and without the need for custom coding. Striim also allows data movement in real-time, because of which stream processing applications need to operate continuously for years. These further speeds up decision-making as insights can be drawn quickly, without latency between receiving the data and running analytics on it.
Check out our case study on real-time data analytics
Case and Point: Simplifying Healthcare Predictions in 3 Expert Steps
Understanding Symptom Patterns: Our first step involves data acquisition and thorough analysis of historical patient data. We tap into the treasure trove of symptoms, medical records, and outcomes to discern intricate patterns that might remain hidden from traditional analysis.
Feature Engineering with Domain Knowledge: With a team of domain experts, we transform raw symptom data into meaningful features. These features are carefully curated to capture the nuances of various symptoms, their interplay, and potential implications. Our domain knowledge empowers us to create a robust feature set that forms the foundation of accurate predictions.
Advanced Machine Learning Models: Equipped with a rich feature set, we employ advanced machine learning models. From ensemble methods to deep learning architectures, we evaluate and fine-tune models that can effectively map symptoms to probable outcomes. This step requires rigorous experimentation to ensure optimal model performance.
The utilization of Symptom Pattern Analysis, Feature Engineering, and Advanced Machine Learning Models in the healthcare domain, along with Indium’s implementation of Striim for real-time data migration and processing, brings substantial and quantifiable business value to the table.
Healthcare Providers: Reduced diagnosis time through rapid predictions – from days to hours, thereby accelerating patient care. Enhanced efficiency with streamlined operations leads to quicker decisions and resource allocation. Improved patient care is achieved through early intervention based on predictions, resulting in improved treatment outcomes. Informed resource allocation provides predictive insights that optimize staff schedules, room usage, and equipment availability. Optimized treatment plans driven by personalized treatments yield better outcomes and patient satisfaction. The Cost savings achieved through fewer hospital stays, reduced redundant tests, and efficient resource use contribute to lowering costs. This not only benefits the patients but also benefits the providers by optimizing their resources.
Healthcare Payers and Insurance Companies: The implementation offers a competitive edge for healthcare providers, attracting patients and enhancing the providers’ reputation due to quick and accurate diagnoses. This, in turn, leads to efficient resource utilization, potentially reducing the overall cost of treatments. Cost savings arising from reduced hospital stays and redundant tests contribute to lower healthcare expenditures, benefiting healthcare payers and insurance companies. Healthcare payers such as insurance companies can also reduce fraudulent claims as they will have access to patient diagnosis history in real-time.
Medical Researchers and Innovators: The curated data fosters research opportunities, facilitating medical insights and potential innovation generation. The advanced analytical capabilities of Symptom Pattern Analysis and Machine Learning Models open avenues for new discoveries and improvements in medical practices, benefiting the broader healthcare research community.
Overall, the integration of advanced technologies, real-time data processing, and predictive analytics in the healthcare domain offers benefits that extend to healthcare providers, payers, patients, and the research community. This synergy drives efficiency, quality of care, and cost-effectiveness, ultimately transforming healthcare delivery and outcomes.
Indium for Instant Decisions with Striim
Indium Software, a cutting-edge solution provider, has deep expertise in Striim implementation and can help businesses create exciting digital experiences for their customers.
A private sector bank offering specialized services to 9 million customers across various business verticals and with a presence global presence required data to be updated in real-time from its core banking systems to a reliable destination database for downstream analytics. By migrating the data from legacy systems to Striim in real time, Indium helped the customer improve its responsiveness and operational efficiency apart from other benefits.
Indium’s team of Striim experts have cross-domain experience and can provide custom-built solutions to meet the unique needs of our customers.
To know more about Indium’s Striim capabilities and solutions
FAQs
The Striim platform offers customers the flexibility to use real-time ETL and ELT on data from multiple sources, including on-prem and cloud databases.
Striim ingests data from major enterprise databases using log-based change data capture (CDC). This lowers the performance load on the database while making data available even before it has been processed.